[Day 5] Understanding of Generative AI
Generative AI goes beyond analysis to create original content—text, images, music, and code—transforming industries and the future of creativity.
Deep learning, a subset of machine learning, is broadly categorized into two types of models: Discriminative and Generative. These models serve different purposes and are foundational to understanding generative AI.
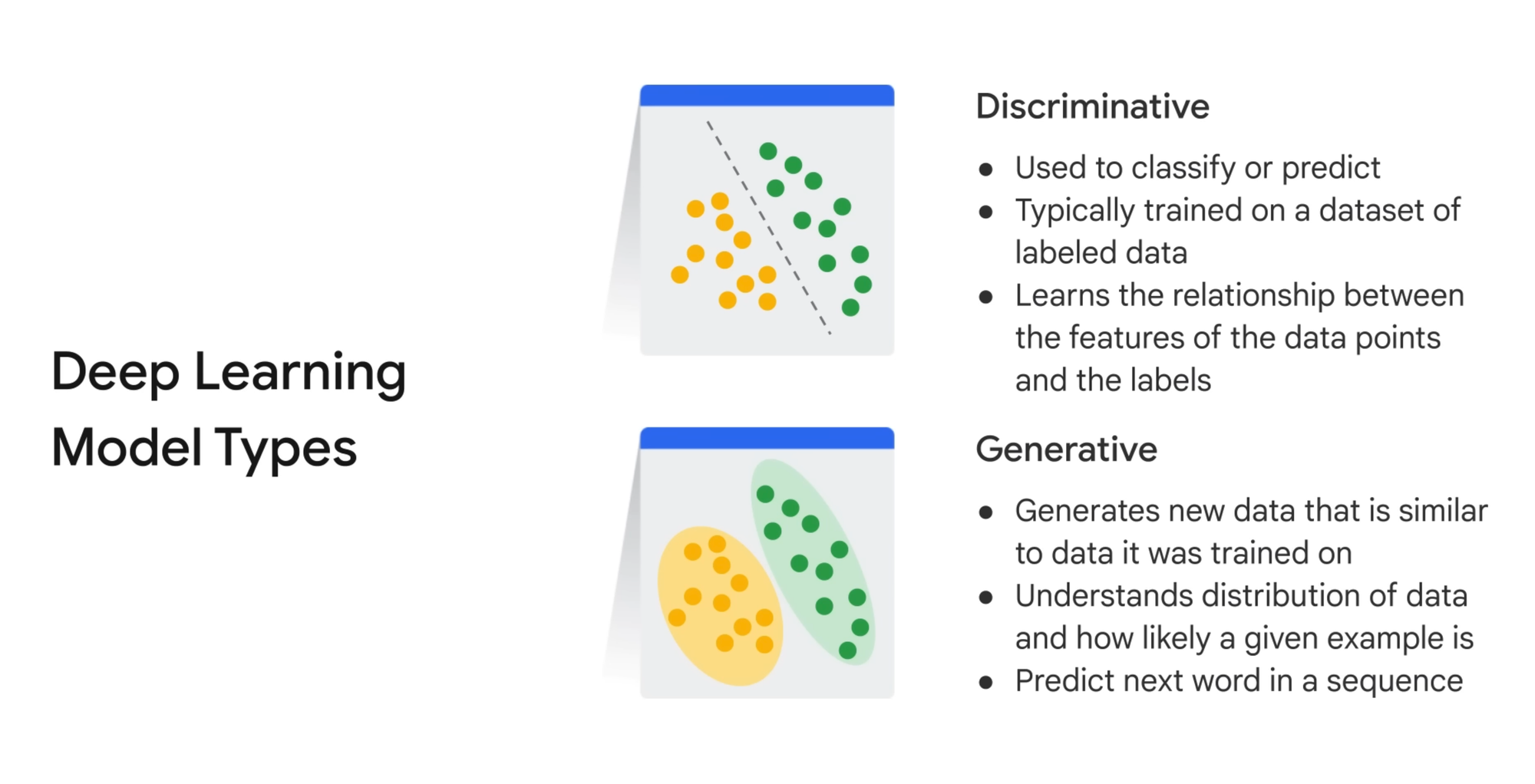
a) Discriminative Models:
Discriminative models focus on distinguishing or classifying data. They predict the probability of a label given an input. For example, a discriminative model might determine whether an image is of a cat or a dog. These models include algorithms like logistic regression, support vector machines, and neural networks used for tasks like classification and regression.
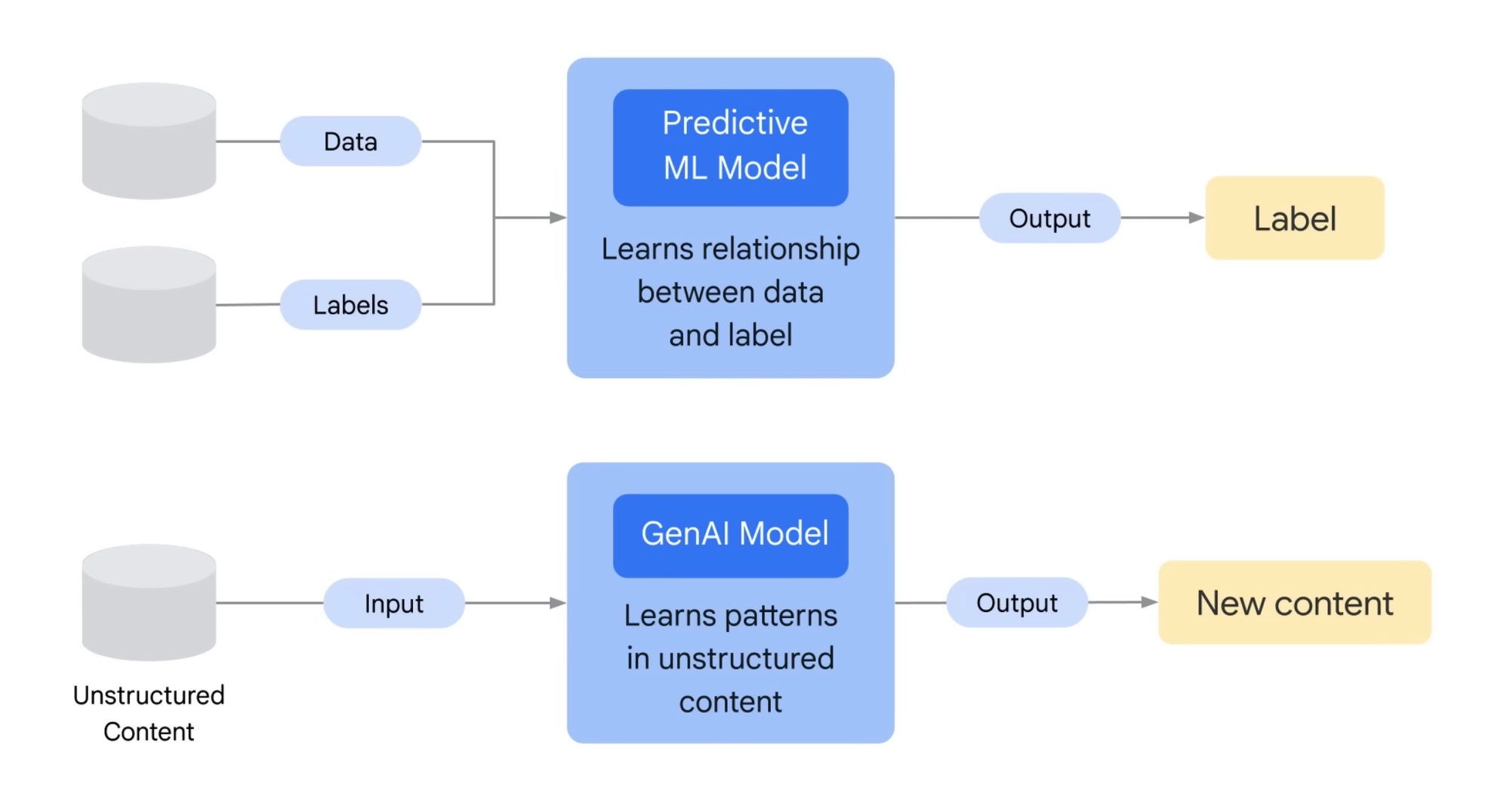
b) Generative Models:
In contrast, generative models aim to understand the underlying distribution of data to generate new data points. Instead of merely classifying inputs, these models can create new content that resembles the data they were trained on. For example, a generative model can produce new images of cats even if those specific images weren’t part of the training data.
Generative AI leverages the power of generative models to produce text, images, music, videos, and more. Let’s dive deeper into generative AI and how it works.
What is Generative AI?
Generative AI is a cutting-edge branch of artificial intelligence that creates new content based on patterns and structures it learns from existing data. Unlike traditional AI, which analyzes and labels data, generative AI generates entirely new outputs, making it a powerful tool for creative and innovative applications.
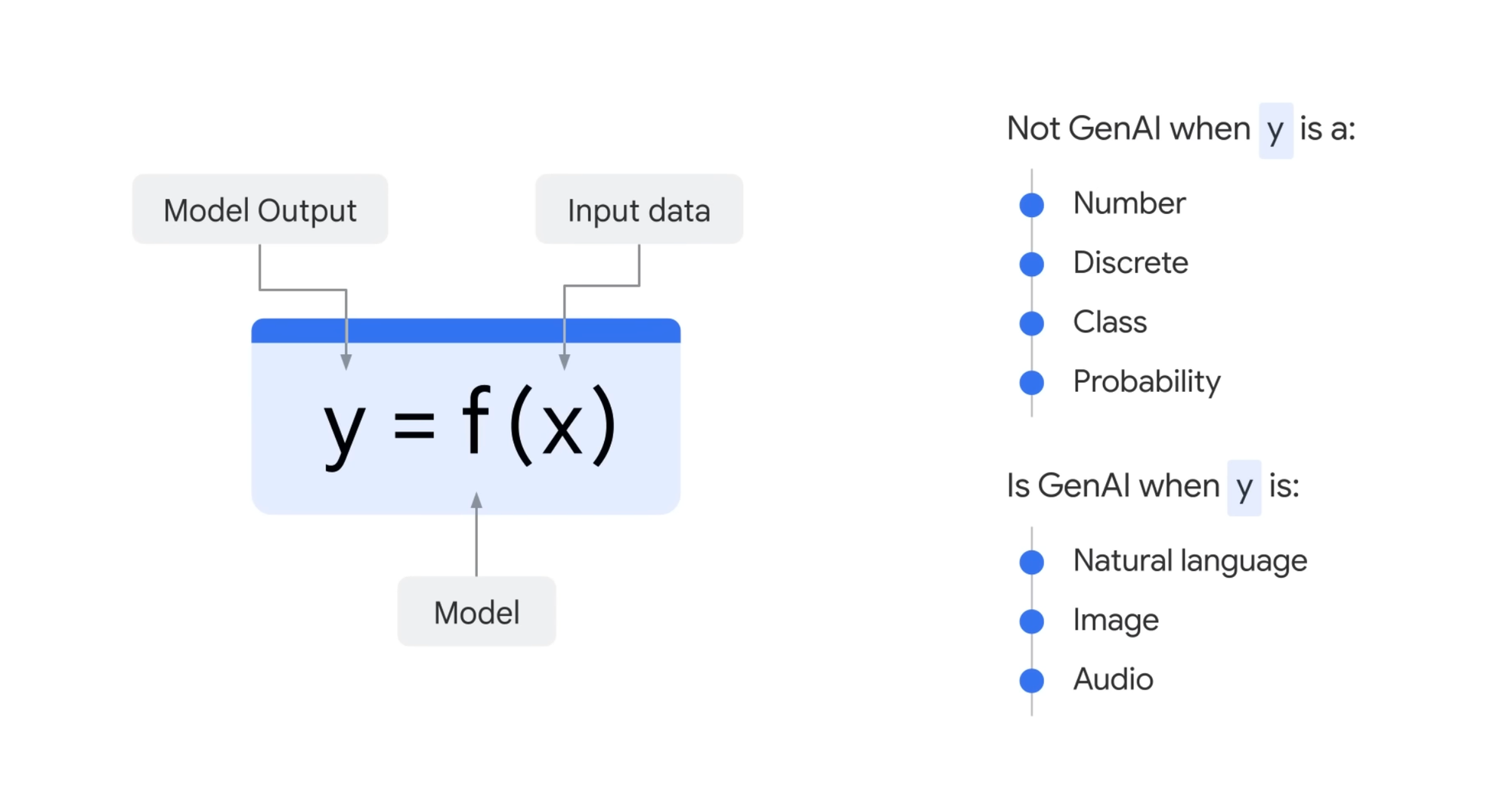
How Does Generative AI Work?
Generative AI relies on advanced deep learning techniques to understand data and generate new content. Here’s a simplified breakdown of the process:
- Training Phase:
- The model is trained on large datasets, analyzing patterns, relationships, and features in the data. For example, a model trained on images learns about shapes, colors, and textures.
- Understanding Latent Space:
- During training, the model creates a compressed representation of the data called a "latent space," which captures the essence of the data distribution.
- Content Generation:
- After training, the model generates new content by sampling from the latent space and reconstructing data based on the learned patterns.
Key Applications of GenAI:
GenAI is rapidly transforming numerous fields:
- Image Generation: Creating photorealistic images, generating artistic renderings, enhancing existing images, and even creating entirely new visual concepts.15 Tools like DALL-E 2, Midjourney, and Stable Diffusion have made this accessible to a wide audience.
- Text Generation: Writing articles, composing poems, generating marketing copy, translating languages, and even writing different kinds of creative content, like emails, letters, code, etc.17 Models like GPT-4 are leading the way in this area.
- Code Generation: Automating the creation of software code, simplifying development processes, and enabling non-programmers to create simple applications.19 GitHub Copilot is a prominent example.
- Music Generation: Composing original music in various styles, creating sound effects, and generating audio for videos and games.
- Video Generation: Creating short videos, generating special effects, and even synthesizing realistic human faces and movements.
- Drug Discovery and Material Science: Designing new molecules and materials with specific properties, accelerating research and development in these fields.
Challenges and Ethical Considerations:
Despite its immense potential, GenAI also raises several challenges and ethical concerns:
- Misinformation and Deepfakes: The ability to generate realistic images and videos can be misused to create convincing fake content, spreading misinformation and manipulating public opinion.
- Copyright and Intellectual Property: Determining ownership and copyright for content generated by AI models is a complex legal issue.
- Bias and Fairness: GenAI models can inherit biases present in their training data, leading to unfair or discriminatory outputs.
- Job Displacement: The automation potential of GenAI raises concerns about potential job displacement in certain industries.
Real-World Examples of Generative AI
1. Text Generation
- Tools: ChatGPT, Jasper AI, Gemini, Perplexity
- Applications: Writing articles, creating scripts, generating chatbot responses.
2. Image and Video Generation
- Tools: DALL-E, Runway ML
- Applications: Creating artwork, enhancing video effects, generating 3D assets.
3. Music Composition
- Tools: AIVA, MuseNet
- Applications: Composing soundtracks, personalizing playlists.
4. Code Generation
- Tools: GitHub Copilot, Codex
- Applications: Assisting developers in writing and debugging code.
5. Healthcare
- Example: Generating synthetic medical data for research, enhancing imaging techniques, or creating personalized treatment plans.
Challenges of Generative AI
- Ethical Concerns: Misuse in creating fake or harmful content.
- Data Requirements: Needs large datasets for effective training.
- Computational Costs: High resource demands for training and deploying models.
- Content Quality: Generated outputs may lack accuracy or reliability.
Nutshell:
Understanding the distinction between discriminative and generative models is essential for appreciating the transformative power of generative AI. From creating stunning visuals to composing music and writing code, generative AI has revolutionized how we approach creativity and problem-solving. As this technology continues to evolve, it offers exciting possibilities while posing challenges that require careful consideration.
Pro Tip for Beginners: Explore free tools like ChatGPT or DALL-E to get hands-on experience with generative AI. Start small, experiment, and unlock your creative potential!
Must watch videos before you move to the next day's article:
- Introduction to Generative AI by Google Cloud Tech -
- Generative AI in a Nutshell - how to survive and thrive in the age of AI by Henrik Kniberg -
💬 Join the DecodeAI WhatsApp Channel for regular AI updates → Click here